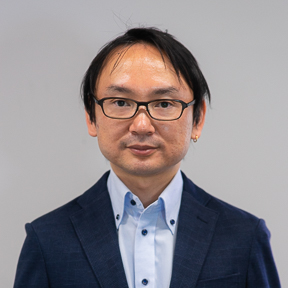
KOBAYASHI Tetsuya Professor
Komaba Campus
Graduate SchoolGraduate School of Engineering - Electrical Engineering and Information Systems
Departmentー
Complex Systems & Bioelectronics Field
Mathematical informatics
Soft computing
Life
System genome science
Biophysics
Artificial Intelligence (AI)
Mathematics and Informatics for Understanding Living Systems
We aim to uncover the design principles of information processing and robustness in biological systems by integrating theoretical and informatics approaches to various biological quantitative data.
Research field 1
Understanding the Principles of Biological Information Processing
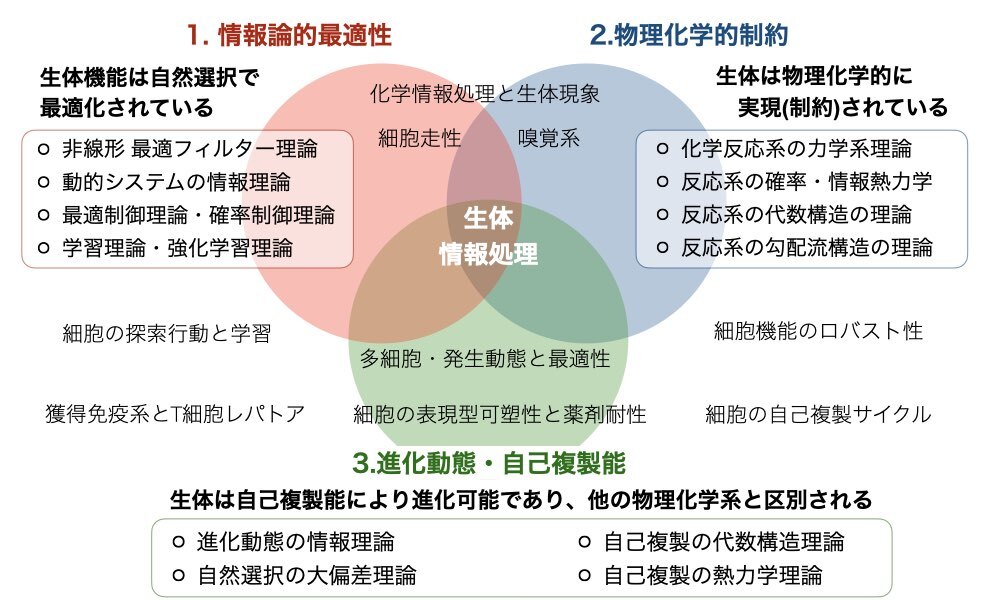
In order to understand the biological information processing systems created by evolution, we focus on three factors: The first is how efficiently the systems are designed (information-theoretic optimality). The second is how the systems are constrained by physical and chemical laws (physico-chemical constraints). The last is how the systems have been realized by balancing the two factors in the evolutionary process (evolvability and self-reproducibility). To unveil the principles of biological information processing, we are developing mathematical and informatic methods to deal with these problems and applying them to various phenomena, including chemical reaction networks, chemotaxis, collective behaviors, immunity, olfaction, and development.
Research field 2
Quantitative Biology and Bioinformatics of Biological Systems and Information Processing
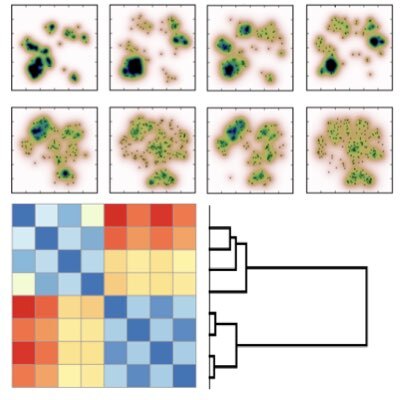
Recent advancements in imaging and sequencing technologies have enabled us to measure the quantitative and dynamical properties of diverse and complex biological systems. We are developing new techniques for quantitative data analysis and bioinformatics being necessary for elucidating various properties of biological systems from such data based on machine learning and deep learning techniques.
Research field 3
Optimality of Biological Information Processing
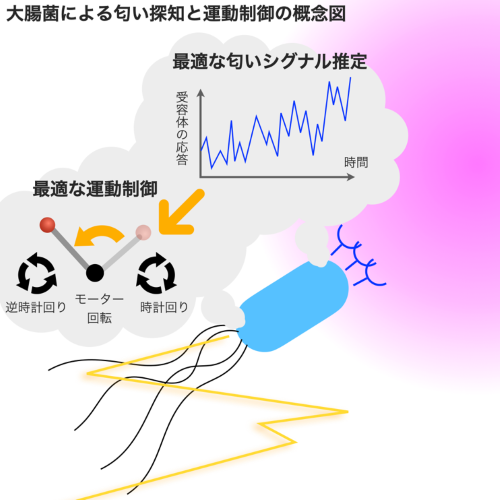
Compared to engineering systems, biological systems have countless aspects that make us think "they are very well designed." However, to answer whether the behavior of living organisms is indeed well designed, it is necessary to understand how close the functions they realize are to being optimal. We are constructing theories to handle the optimality of biological functions and information processing by integrating optimization theories such as information theory and control theory, along with physical theories like non-equilibrium thermodynamics. Furthermore, we are verifying the optimality of living organisms by comparing these theoretical results with quantitative measurements in various biological systems, including the immune system and olfactory system.
Research field 4
Biological Functions and Nonequilibrium Network
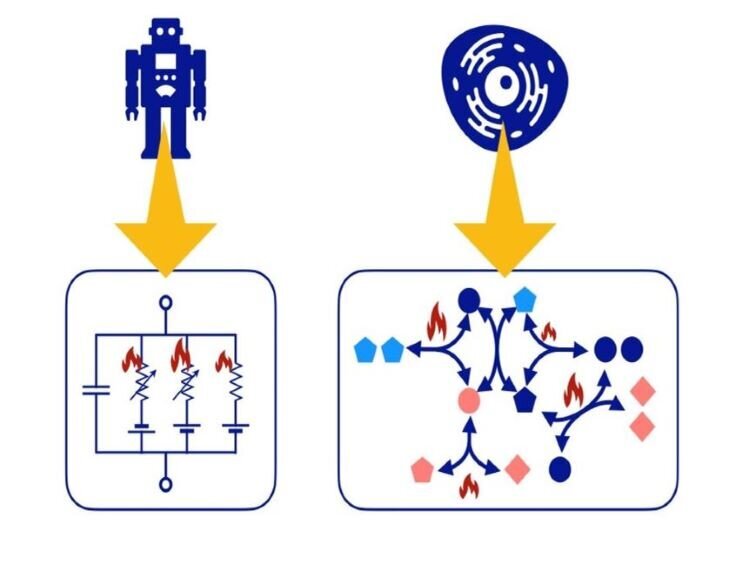
Just as engineering systems are made up of electrical circuits and machinery, biological cells, the building blocks of biological systems, are composed of networks (circuits) of chemical reactions. The network structure determines the functions of biological systems, and the dynamics of networks being generally non-equilibrium are strongly constrained by physicochemical laws and non-equilibrium thermodynamic characteristics. We are developing methods that combine network theory with non-equilibrium thermodynamics to understand how the functions and possible behaviors of biological systems are constrained by the laws of physics and chemistry.
Research field 5
Integrative Understanding of Learning and Evolution for Biological Adaptations
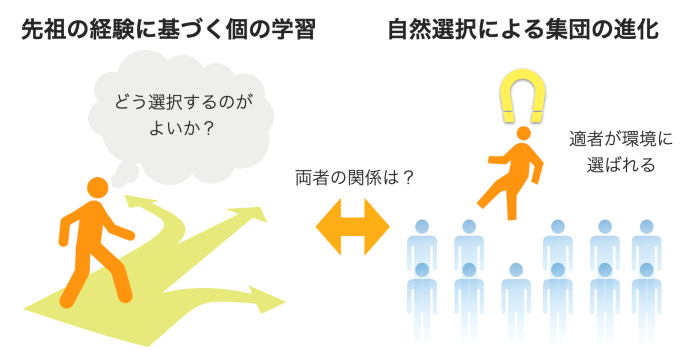
Biological systems possess the ability to adapt flexibly to ever-changing environment. Darwinian evolution, based on natural selection, is one of the fundamental mechanisms for adaptation. On the other hand, biological systems have developed organs such as the brain that actively sense and predict environmental changes, allowing them to choose adaptive states in advance. How are these two adaptive mechanisms related?
We are working on the construction and application of a unified theory of adaptation in living organisms by theoretically integrating these two adaptive mechanisms using the information-theoretic variational structure common to both Darwinian natural selection and predictive information processing.