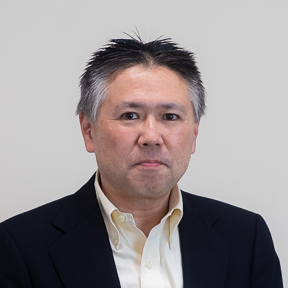
TAKEUCHI Ken Professor
Hongo Campus
Systems & Electronics
Computer Systems
High-Performance Computing
Perceptual information processing
Intelligent informatics
Electron device/Electronic equipment
Data Centric Computing (AI / Computation in Memory / Quantum Computer)
Takeuchi Lab is extensively studying brain-like data-centric computing and Computation in memory (CiM) that combines data processing and memory. We foster students who understand application and social implementation and then co-design different fields from LSI hardware, software, and machine learning for AI era.
Research field 1
Computation of event sensing data
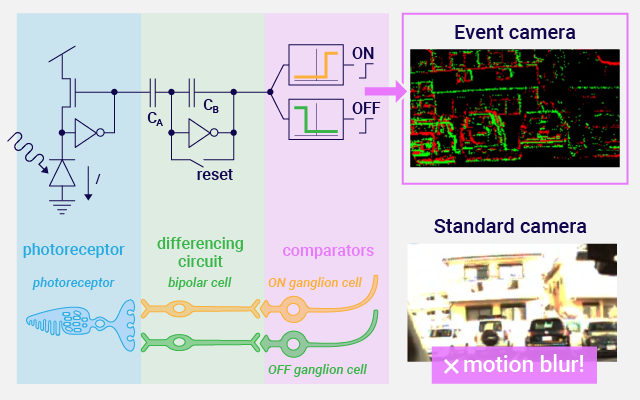
Like human retina, an event camera asynchronously outputs event signal when the logarithmic intensity of light changes. The pixel of event camera is composed of a photo diode, differential circuit, and comparator, and thus have high dynamic range. We are researching neuromorphic circuit systems that can reduce redundant data processing and process data at high speed and low power by detecting/calculating data only when an event occurs. With traditional frame-based cameras, points moving between frames cause motion blur. On the other hand, in our research, motion blur is eliminated by processing event data quickly. We are aiming that moving objects such as self-driving cars and drones move autonomously, safely and securely by recognizing the environment and objects accurately at high speed.
Research field 2
Computation in memory
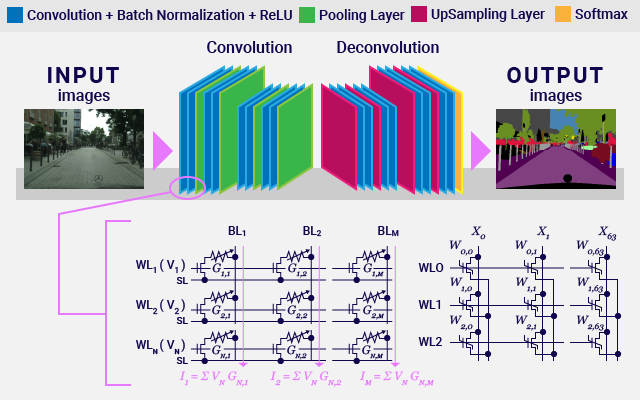
Autonomous driving application performs a huge number of multiply-accumulation (MAC) for image and object recognition. Takeuchi Lab conducts research on CiM suitable for deep learning. Non-volatile memories such as Resistive RAM (ReRAM) and Ferroelectric FET (FeFET) store weights of neural networks. Inference of deep learning is executed at high speed by driving non-volatile memory cell arrays simultaneously in massively parallel.
Research field 3
Ai chip
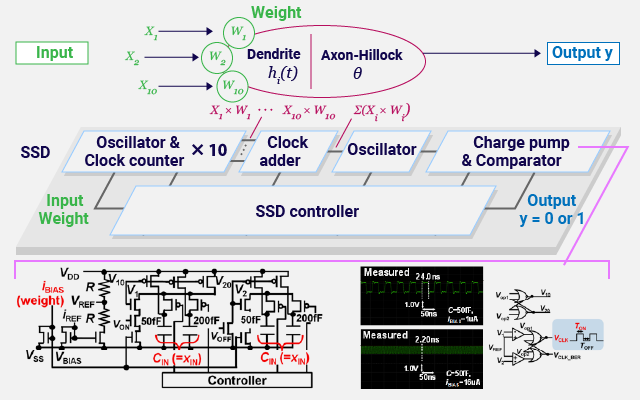
We are studying processors, called AI chip, which executes machine learning at high speed and low power. One example is neuromorphic computing chip which mimics human neurons by using analog circuits. Up to now, time-domain multiply accumulation (MAC) operation is realized by flexibly controlling the on/off time of the novel oscillation circuit according to the inputs and the weights of neural network. The proposed MAC operation improves power efficiency by about 10 times.
Research field 4
Approximate computing
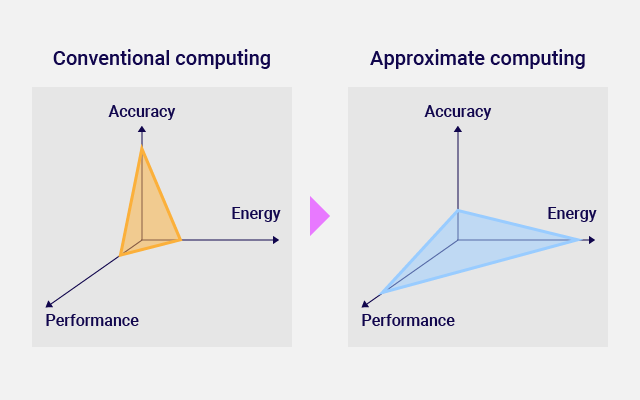
Processors and memories in computers have trade-offs among performance, power, cost (price) and reliability. Conventional computing does not allow errors because of the sequential processing of executing programs. On the other hand, statistical machine learning applications like image recognition and speech recognition tolerate some errors and inaccuracies. Naturally, human recognition is not perfect. We are working on Approximate computing for machine learning that tolerates errors and inaccuracies at the LSI level such as processor and memory. In the application level, on the other hand, machine learning performs recognition accurately in the same way as conventional computing. Toward future AI applications, we are researching Domain-specific computing optimized for each application.
Research field 5
Brain-inspired memory
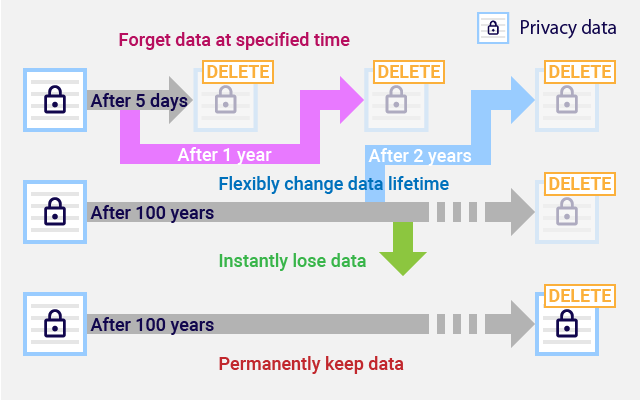
Important data are stored forever, and secret privacy data are forgotten at appropriate time. The brain-inspired memory can flexibly specify the lifetime of data, and extend or shorten the lifetime of data while the data are stored in memory. Considering the privacy such as the right to be forgotten, sensitive personal data can be permanently erased. We realize the protection of privacy data by intelligent hardware technology, that cannot be solved by software alone.